Received: Wed 04, Dec 2024
Accepted: Thu 19, Dec 2024
Abstract
Background: Colorectal cancer (CRC) is a leading cause of mortality in China, with metastasis significantly contributing to poor outcomes. Histopathological growth patterns (HGPs) in colorectal liver metastasis (CRLM) provide vital prognostic insights, yet the limited number of pathologists highlights the need for auxiliary diagnostic tools. Recent advancements in artificial intelligence (AI) have demonstrated potential in enhancing diagnostic precision, prompting the development of specialized AI models like COFFEE to improve the classification and management of HGPs in CRLM patients.
Methods: This study developed a transformer-based deep learning model, COFFEE, for the precise classification of colorectal cancer subtypes using whole slide images (WSIs) from 514 patients diagnosed with colorectal cancer liver metastasis. The model was pre-trained using DINO on 1,442 WSIs from the TCGA-COAD cohort, utilizing a vision transformer (ViT) architecture to extract 384-dimensional feature vectors from 256 × 256 pixel patches. The proposed model integrates a transformer-based multiple instance learning (TransMIL) framework, which effectively aggregates spatial and morphological information through multi-head self-attention and pyramid position encoding generator (PPEG) modules. This design enables efficient handling of large instance sequences within WSIs, allowing for accurate binary and four-class classification. The model was validated on 972 WSIs from a recent dataset, demonstrating its robustness and clinical applicability.
Results: A total of 431 patients were included in three cohorts: training (n=297), testing (n=104), and prospective (n=30). Desmoplastic tumors were associated with longer overall survival (OS, 53.6 vs. 31.9 months, p=0.002) and progression-free survival (PFS, 25.2 vs. 10.7 months, p<0.001) compared to non-desmoplastic tumors. The COFFEE binary classification model achieved high predictive performance with AUC values of 0.961 in the training, 0.935 in the testing, and 1.000 in the prospective cohort. The four-class model also showed strong performance, with AUCs of 0.961 and 0.966 in the training and testing cohorts, and 0.985 in the prospective cohort. AI-assisted models helped junior pathologists achieve an accuracy of 94.7% (vs. 85.9%) and reduced diagnostic time by 36%, improving both accuracy and speed.
Conclusion: This study developed the first AI model for HGP classification in colorectal cancer liver metastasis, achieving high accuracy in both binary classification and four-class classification models. The model demonstrated potential for improving diagnostic precision and guiding post-surgery treatment strategies, with AI-assisted pathologists surpassing traditional methods in a prospective randomized trial.
Keywords
Colorectal liver metastasis (CRLM), histopathological growth patterns (HGPs), artificial intelligence (AI) in diagnosis, vision transformer (ViT), desmoplastic classification
TABLE 1. Baseline
characteristics of training, testing, and prospective cohorts.
Variable |
Training cohort (N = 297) |
Testing cohort (N = 104) |
Prospective cohort (N = 30) |
Follow up, months (median, IQR) |
23 (16, 38) |
11 (8, 17) |
6 (5, 7) |
Gender |
|
|
|
Female |
89 (30%) |
42 (40%) |
14 (47%) |
Male |
208 (70%) |
62 (60%) |
16 (53%) |
Age, years (median, IQR) |
58 (49, 65) |
58 (51, 65) |
56 (42, 61) |
<60 |
167 (56%) |
59 (57%) |
17 (57%) |
≥60 |
130 (44%) |
45 (43%) |
13 (43%) |
CEA (U/ml, [median, IQR]) |
7 (3, 21) |
7 (4, 21) |
5 (3, 19) |
CA199 (U/ml, [median, IQR]) |
12 (5, 59) |
15 (5, 75) |
9 (5, 37) |
CA125 (U/ml, [median, IQR]) |
13 (9, 19) |
12 (8, 19) |
14 (10, 21) |
Number of liver segments involved |
|
|
|
≤2 |
169 (57%) |
48 (46%) |
14 (47%) |
3 |
56 (19%) |
19 (18%) |
3 (10%) |
4 |
37 (12%) |
12 (12%) |
5 (17%) |
≥5 |
35 (12%) |
25 (24%) |
8 (27%) |
Number of liver metastases |
|
|
|
≤2 |
175 (59%) |
53 (51%) |
14 (47%) |
3 - 5 |
70 (24%) |
23 (22%) |
5 (17%) |
≥5 |
52 (18%) |
28 (27%) |
11 (37%) |
Maximum size of liver metastases exceeds 3cm |
|
|
|
No |
148 (50%) |
65 (63%) |
23 (77%) |
Yes |
149 (50%) |
39 (38%) |
7 (23%) |
Preoperative chemotherapy |
|
|
|
No |
142 (48%) |
38 (37%) |
7 (23%) |
Yes |
155 (52%) |
66 (63%) |
23 (77%) |
Tumor site |
|
|
|
Left colon |
244 (82%) |
68 (65%) |
26 (87%) |
Right colon |
53 (18%) |
36 (35%) |
4 (13%) |
Pathological T stage |
|
|
|
T0 |
6 (2.0%) |
0 (0%) |
1 (3.3%) |
T1 |
2 (0.7%) |
0 (0%) |
0 (0%) |
T2 |
27 (9.1%) |
8 (7.7%) |
3 (10%) |
T3 |
197 (66%) |
73 (70%) |
24 (80%) |
T4 |
65 (22%) |
23 (22%) |
2 (6.7%) |
Pathological N stage |
|
|
|
N0 |
102 (34%) |
43 (42%) |
13 (43%) |
N1 |
146 (49%) |
38 (37%) |
13 (43%) |
N2 |
48 (16%) |
22 (21%) |
4 (13%) |
Pathological type |
|
|
|
Infiltrating |
45 (15%) |
20 (19%) |
3 (10%) |
Mass |
89 (30%) |
23 (22%) |
6 (20%) |
Ulcerative |
163 (55%) |
61 (59%) |
21 (70%) |
Differentiation |
|
|
|
Highly |
39 (13%) |
9 (8.7%) |
2 (6.7%) |
Moderately |
215 (72%) |
80 (77%) |
27 (90%) |
Poorly |
43 (14%) |
15 (14%) |
1 (3.3%) |
Intravascular tumor thrombus |
|
|
|
No |
204 (69%) |
64 (62%) |
22 (73%) |
Yes |
93 (31%) |
40 (38%) |
8 (27%) |
Ki67 |
50 (30, 70) |
60 (40, 70) |
70 (40, 70) |
HER2 stage* |
|
|
|
0 |
213 (72%) |
80 (78%) |
22 (73%) |
1+ |
49 (16%) |
18 (17%) |
6 (20%) |
2+ |
23 (7.7%) |
3 (2.9%) |
2 (6.7%) |
3+ |
12 (4.0%) |
2 (1.9%) |
0 (0%) |
Genes mutation |
|
|
|
Wild type |
145 (49%) |
62 (62%) |
17 (57%) |
Mutation** |
152 (51%) |
38 (38%) |
13 (43%) |
BRAF mutation |
23 (7.6%) |
3 (2.9%) |
2 (6.3%) |
EGFR mutation |
1 (0.3%) |
1 (1.0%) |
0 (0%) |
KRAS mutation |
71 (24%) |
25 (24%) |
11 (34%) |
NRAS mutation |
28 (9.3%) |
1 (1.0%) |
0 (0%) |
PIK3CA mutation |
34 (11%) |
11 (11%) |
2 (6.3%) |
UGT1A1 mutation |
0 (0%) |
1 (1.0%) |
0 (0%) |
HER2: Human Epidermal growth factor receptor 2; CEA: Carcinoembryonic
Antigen; CA199: Carbohydrate Antigen 19-9; CA125: Cancer Antigen 125; IQR: Interquartile
Range.
* 0 (Negative): No membrane positivity, 0% proportion; interpreted as
negative;
1+ (Weakly Positive): Weak membrane positivity, ≤10% proportion;
interpreted as negative;
2+ (Equivocal): Moderate to strong membrane positivity, 10-50% or ≥50%
proportion; interpreted as equivocal, FISH testing recommended;
3+ (Positive): Strong membrane positivity, ≥50% proportion; interpreted
as positive.
** Eleven patients have double gene mutations.
TABLE 2. Pathological
classifications in training testing and, prospective cohorts.
Variable |
Training cohort (N = 297) |
Testing cohort (N = 104) |
Prospective cohort (N = 30) |
Binary
pathological classification |
|||
Desmoplastic |
98 (33%) |
39 (38%) |
7 (23%) |
Non-desmoplastic |
199 (67%) |
65 (63%) |
23 (77%) |
Four-class pathological classification |
|||
Desmoplastic |
223 (75%) |
75 (72%) |
20 (67%) |
Replacement |
42 (14%) |
12 (12%) |
7 (23%) |
Pushing |
21 (7.1%) |
11 (11%) |
0 (0%) |
Mixed |
11 (3.7%) |
6 (5.8%) |
3 (10%) |
TABLE 3. Clinicopathological characteristics of the training cohort based on
binary pathological classification.
Variable |
Desmoplastic |
Non-desmoplastic |
p-value |
Gender |
|
|
0.2 |
Female |
25 (26%) |
64 (32%) |
|
Male |
73 (74%) |
135 (68%) |
|
Age, years (median, IQR) |
58 (47, 64) |
58 (49, 66) |
0.4 |
<60 |
59 (60%) |
108 (54%) |
0.3 |
≥60 |
39 (40%) |
91 (46%) |
|
CEA (U/ml, [median, IQR]) |
6 (3, 12) |
9 (4, 29) |
0.002 |
CA199 (U/ml, [median, IQR]) |
8 (4, 25) |
18 (6, 90) |
0.002 |
CA125 (U/ml, [median, IQR]) |
12 (9, 21) |
13 (8, 19) |
0.6 |
Number of liver segments involved |
|
|
0.7 |
≤2 |
57 (58%) |
112 (56%) |
|
3 |
21 (21%) |
35 (18%) |
|
4 |
10 (10%) |
27 (14%) |
|
≥5 |
10 (10%) |
25 (13%) |
|
Number of liver metastases |
|
|
0.6 |
≤2 |
61 (62%) |
114 (57%) |
|
3 - 5 |
20 (20%) |
50 (25%) |
|
≥5 |
17 (17%) |
35 (18%) |
|
Maximum size of liver metastases exceeds 3cm |
|
|
0.7 |
No |
47 (48%) |
101 (51%) |
|
Yes |
51 (52%) |
98 (49%) |
|
Preoperative chemotherapy |
|
|
0.8 |
No |
46 (47%) |
96 (48%) |
|
Yes |
52 (53%) |
103 (52%) |
|
Tumor site |
|
|
0.036 |
Left colon |
74 (76%) |
170 (85%) |
|
Right colon |
24 (24%) |
29 (15%) |
|
Pathological T stage |
|
|
0.3 |
T0 |
4 (4.1%) |
2 (1.0%) |
|
T1 |
0 (0%) |
2 (1.0%) |
|
T2 |
11 (11%) |
16 (8.0%) |
|
T3 |
63 (64%) |
134 (67%) |
|
T4 |
20 (20%) |
45 (23%) |
|
Pathological N stage |
|
|
0.061 |
N0 |
42 (43%) |
60 (30%) |
|
N1 |
45 (46%) |
101 (51%) |
|
N2 |
11 (11%) |
37 (19%) |
|
Pathological type |
|
|
0.2 |
Infiltrating |
18 (18%) |
27 (14%) |
|
Mass |
33 (34%) |
56 (28%) |
|
Ulcerative |
47 (48%) |
116 (58%) |
|
Differentiation |
|
|
0.4 |
Highly |
16 (16%) |
23 (12%) |
|
Moderately |
70 (71%) |
145 (73%) |
|
Poorly |
12 (12%) |
31 (16%) |
|
Intravascular tumor thrombus |
|
|
0.9 |
No |
68 (69%) |
136 (68%) |
|
Yes |
30 (31%) |
63 (32%) |
|
Ki67 |
50 (30, 70) |
50 (30, 70) |
0.6 |
HER2 stage* |
|
|
0.6 |
0 |
71 (72%) |
142 (71%) |
|
1+ |
13 (13%) |
36 (18%) |
|
2+ |
9 (9.2%) |
14 (7.0%) |
|
3+ |
5 (5.1%) |
7 (3.5%) |
|
Gene mutation |
|
|
0.4 |
Wild type |
51 (52%) |
94 (47%) |
|
Mutation** |
47 (48%) |
105 (53%) |
|
BRAF mutation |
9 (9.2%) |
14 (6.9%) |
|
EGFR mutation |
1 (1.0%) |
0 (0%) |
|
KRAS mutation |
20 (20%) |
51 (25%) |
|
NRAS mutation |
8 (8.2%) |
20 (9.8%) |
|
PIK3CA mutation |
9 (9.2%) |
25 (12%) |
|
Median OS, months (95% CI) |
53.6 (45.5-NA) |
31.9 (27.8-45.1) |
0.002 |
Median PFS,
months (95% CI) |
25.2 (18.10-38.3) |
10.7 (8.07-13.6) |
<0.001 |
HER2: Human Epidermal Growth Factor Receptor 2; CEA: Carcinoembryonic
Antigen; CA199: Carbohydrate Antigen 19-9; CA125: Cancer Antigen 125; IQR:
Interquartile Range; OS: overall survival; PFS: Progression-Free Survival.
* 0 (Negative): No membrane positivity, 0% proportion; interpreted as
negative;
1+ (Weakly Positive): Weak membrane positivity, ≤10% proportion;
interpreted as negative;
2+ (Equivocal): Moderate to strong membrane positivity, 10-50% or ≥50%
proportion; interpreted as equivocal, FISH testing recommended;
3+ (Positive): Strong membrane positivity, ≥50% proportion; interpreted
as positive.
** Five patients have double gene mutations.
TABLE 4. Clinicopathological
characteristics of the training cohort based on four-class pathological
classification.
Variable |
Desmoplastic |
Replacement |
Pushing |
Mixed |
p-value |
Gender |
|
|
|
|
0.2 |
Female |
62 (28%) |
15 (36%) |
10 (48%) |
2 (18%) |
|
Male |
161 (72%) |
27 (64%) |
11 (52%) |
9 (82%) |
|
Age, years (median, IQR) |
58 (50, 66) |
54 (47, 64) |
61 (56, 66) |
60 (44, 67) |
0.4 |
<60 |
127 (57%) |
26 (62%) |
9 (43%) |
5 (45%) |
0.4 |
≥60 |
96 (43%) |
16 (38%) |
12 (57%) |
6 (55%) |
|
CEA (U/ml, [median, IQR]) |
6 (3, 19) |
12 (5, 38) |
10 (4, 41) |
18 (9, 98) |
0.006 |
CA199 (U/ml, [median, IQR]) |
10 (5, 38) |
40 (9, 147) |
15 (5, 171) |
38 (9, 255) |
0.008 |
CA125 (U/ml, [median, IQR]) |
12 (9, 19) |
14 (9, 19) |
12 (9, 17) |
17 (9, 24) |
0.6 |
Number of liver segments involved |
|
|
|
|
0.94 |
≤2 |
126 (57%) |
23 (55%) |
12 (57%) |
8 (73%) |
|
3 |
43 (19%) |
9 (21%) |
3 (14%) |
1 (9.1%) |
|
4 |
29 (13%) |
5 (12%) |
3 (14%) |
0 (0%) |
|
≥5 |
25 (11%) |
5 (12%) |
3 (14%) |
2 (18%) |
|
Number of liver metastases |
|
|
|
|
0.8 |
≤2 |
132 (59%) |
24 (57%) |
13 (62%) |
6 (55%) |
|
3 - 5 |
55 (25%) |
8 (19%) |
5 (24%) |
2 (18%) |
|
≥5 |
36 (16%) |
10 (24%) |
3 (14%) |
3 (27%) |
|
Maximum size of liver metastases exceeds 3cm |
|
|
|
|
0.6 |
No |
107 (48%) |
24 (57%) |
12 (57%) |
5 (45%) |
|
Yes |
116 (52%) |
18 (43%) |
9 (43%) |
6 (55%) |
|
Preoperative chemotherapy |
|
|
|
|
0.6 |
No |
108 (48%) |
18 (43%) |
12 (57%) |
4 (36%) |
|
Yes |
115 (52%) |
24 (57%) |
9 (43%) |
7 (64%) |
|
Tumor site |
|
|
|
|
0.4 |
Left colon |
179 (80%) |
38 (90%) |
17 (81%) |
10 (91%) |
|
Right colon |
44 (20%) |
4 (9.5%) |
4 (19%) |
1 (9.1%) |
|
Pathological T stage |
|
|
|
|
0.99 |
T0 |
5 (2.2%) |
1 (2.4%) |
0 (0%) |
0 (0%) |
|
T1 |
1 (0.4%) |
1 (2.4%) |
0 (0%) |
0 (0%) |
|
T2 |
21 (9.4%) |
3 (7.1%) |
2 (9.5%) |
1 (9.1%) |
|
T3 |
148 (66%) |
27 (64%) |
15 (71%) |
7 (64%) |
|
T4 |
48 (22%) |
10 (24%) |
4 (19%) |
3 (27%) |
|
Pathological N stage |
|
|
|
|
0.3 |
N0 |
80 (36%) |
11 (26%) |
8 (38%) |
3 (27%) |
|
N1 |
108 (49%) |
23 (55%) |
7 (33%) |
8 (73%) |
|
N2 |
34 (15%) |
8 (19%) |
6 (29%) |
0 (0%) |
|
Pathological type |
|
|
|
|
0.12 |
Infiltrating |
32 (14%) |
5 (12%) |
4 (19%) |
4 (36%) |
|
Mass |
75 (34%) |
9 (21%) |
3 (14%) |
2 (18%) |
|
Ulcerative |
116 (52%) |
28 (67%) |
14 (67%) |
5 (45%) |
|
Differentiation |
|
|
|
|
0.5 |
Highly |
31 (14%) |
5 (12%) |
3 (14%) |
0 (0%) |
|
Moderately |
162 (73%) |
30 (71%) |
16 (76%) |
7 (64%) |
|
Poorly |
30 (13%) |
7 (17%) |
2 (9.5%) |
4 (36%) |
|
Intravascular tumor thrombus |
|
|
|
|
0.6 |
No |
153 (69%) |
27 (64%) |
17 (81%) |
7 (64%) |
|
Yes |
70 (31%) |
15 (36%) |
4 (19%) |
4 (36%) |
|
Ki67 |
50 (30, 70) |
50 (30, 70) |
40 (30, 70) |
40 (20, 70) |
0.7 |
HER2 stage* |
|
|
|
|
0.019 |
0 |
161 (72%) |
32 (76%) |
15 (71%) |
5 (45%) |
|
1+ |
37 (17%) |
7 (17%) |
3 (14%) |
2 (18%) |
|
2+ |
16 (7.2%) |
3 (7.1%) |
3 (14%) |
1 (9.1%) |
|
3+ |
9 (4.0%) |
0 (0%) |
0 (0%) |
3 (27%) |
|
Gene mutation |
|
|
|
|
0.6 |
Wild type |
106 (48%) |
23 (55%) |
12 (57%) |
4 (36%) |
|
Mutation** |
117 (52%) |
19 (45%) |
9 (43%) |
7 (64%) |
|
BRAF mutation |
17 (7.5%) |
6 (14%) |
0 (0%) |
0 (0%) |
|
EGFR mutation |
1 (0.4%) |
0 (0%) |
0 (0%) |
0 (0%) |
|
KRAS mutation |
54 (24%) |
7 (16%) |
6 (29%) |
4 (36%) |
|
NRAS mutation |
22 (9.7%) |
4 (9.3%) |
2 (9.5%) |
0 (0%) |
|
PIK3CA mutation |
27 (12%) |
3 (7.0%) |
1 (4.8%) |
3 (27%) |
|
Median OS, months (95% CI) |
51.0 (37.9-73.7) |
26.4 (22.1-NA) |
58.3 (28.3-NA) |
20.0 (18.2-NA) |
0.033 |
Median PFS,
months (95% CI) |
17.38 (14.72-20.9) |
7.98 (5.48-12.2) |
12.20 (5.15-34.2) |
6.82 (5.21-NA) |
<0.001 |
HER2: Human Epidermal Growth Factor Receptor 2; CEA: Carcinoembryonic
Antigen; CA199: Carbohydrate Antigen 19-9; CA125: Cancer Antigen 125; IQR: Interquartile
Range; OS: Overall Survival; PFS: Progression-Free Survival.
* 0 (Negative): No membrane positivity, 0% proportion; interpreted as
negative;
1+ (Weakly Positive): Weak membrane positivity, ≤10% proportion;
interpreted as negative;
2+ (Equivocal): Moderate to strong membrane positivity, 10-50% or ≥50%
proportion; interpreted as equivocal, FISH testing recommended;
3+ (Positive): Strong membrane positivity, ≥50% proportion; interpreted
as positive.
** Five patients have double gene mutations.
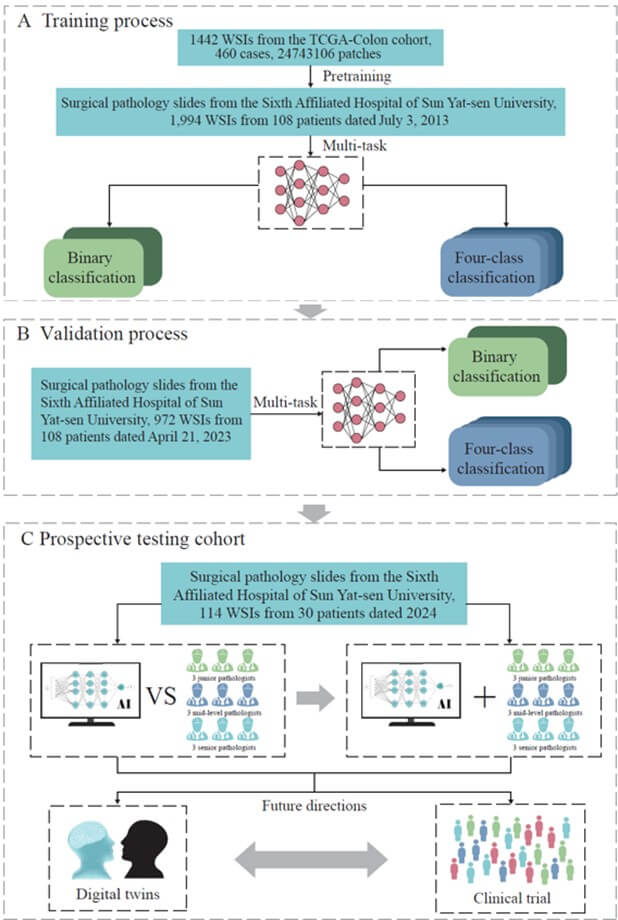
A) Training process: The model was pre-trained using the TCGA-Colon cohort, followed by further training with CRLM pathology slides from SAHSYSU (2013). The model demonstrated high accuracy and speed in binary and four-class classifications, aiding pathologists with rapid diagnostic results. B) Testing process: The COFFEE model was tested using 2023 CRLM pathology slides from SAHSYSU. Results from data collected a decade earlier confirmed the model’s reliability in clinical practice. C) Prospective validation cohort: In 2024, pathology slides from 30 CRLM patients were used to evaluate the COFFEE model. The left framework compared the model’s performance with that of junior, intermediate, and senior pathologists in binary and four-class classifications. The right framework assessed the impact of COFFEE model assistance on pathologist performance. The results showed that the COFFEE model achieved comparable accuracy to senior pathologists with faster classification speeds, significantly enhancing the accuracy and speed of pathologists in WSI-based CRLM classification. The model also has potential for future applications in digital twin technology and clinical trials.
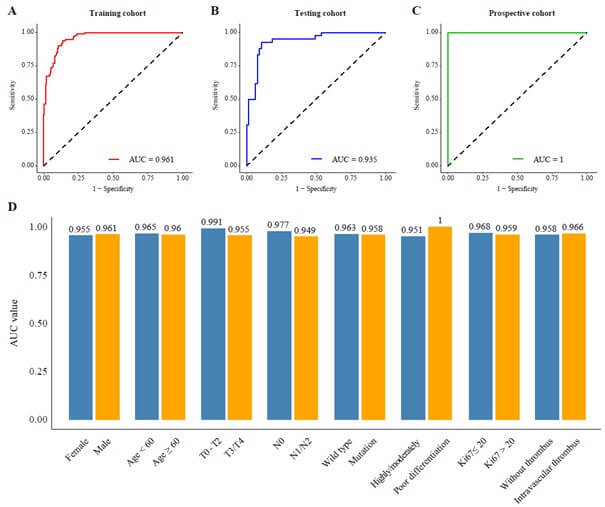
Performance in A) the training cohort, B) the testing cohort, and C) the prospective cohort, D) subgroup analysis of AUC values.
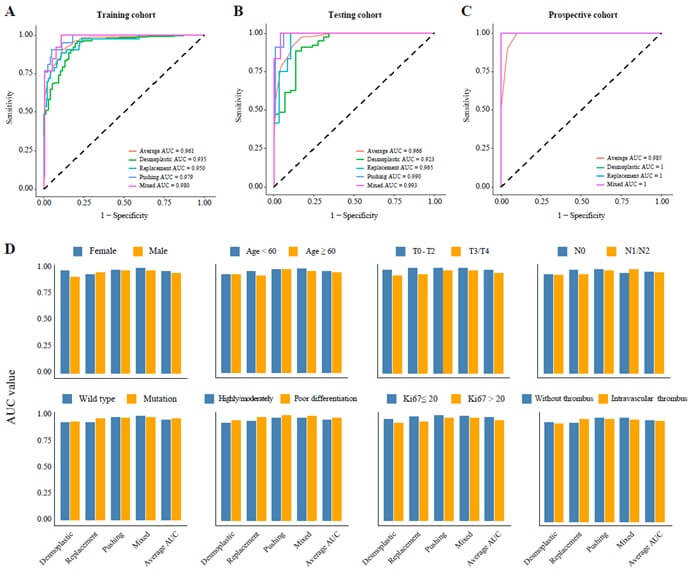
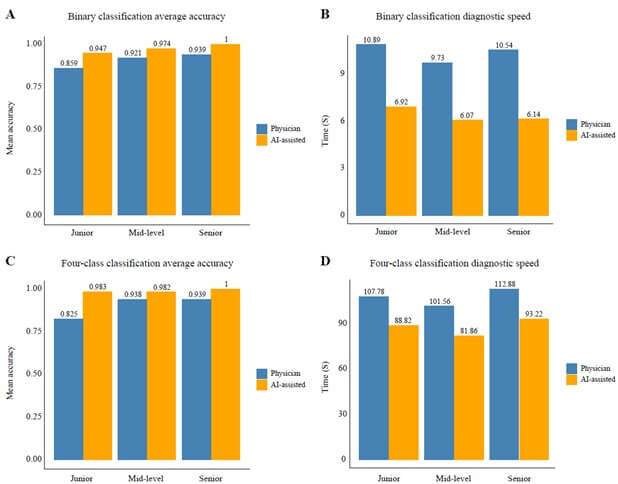
REFERENCES
1. Rebecca L Siegel, Angela N
Giaquinto, Ahmedin Jemal “Cancer statistics, 2024.” CA Cancer J Clin,
vol. 74, no, 1, pp. 12-49, 2024. Erratum in: CA Cancer J Clin, vol. 74,
no. 2, pp. 203, 2024. View at: Publisher Site | PubMed
2. Ranmali Ranasinghe, Michael Mathai,
Anthony Zulli “A synopsis of modern - day colorectal cancer: Where we stand.” Biochim
Biophys Acta Rev Cancer, vol. 1877, no. 2, pp. 188699, 2022. View at: Publisher
Site
| PubMed
3. René Adam, Aimery De Gramont, Joan
Figueras, et al. “The oncosurgery approach to managing liver metastases from
colorectal cancer: a multidisciplinary international consensus.” Oncologist,
vol. 17, no. 10, pp. 1225-1239, 2012. View at: Publisher
Site
| PubMed
4. L Viganò, B Branciforte, V Laurenti,
et al. “The Histopathological Growth Pattern of Colorectal Liver Metastases
Impacts Local Recurrence Risk and the Adequate Width of the Surgical Margin.” Ann
Surg Oncol, vol. 29, no. 9, pp. 5515-5524, 2022. View at: Publisher
Site
| PubMed
5. Pieter-Jan van Dam, Sofie Daelemans,
Elizabeth Ross, et al. “Histopathological growth patterns as a candidate
biomarker for immunomodulatory therapy.” Semin Cancer Biol, vol. 52, no.
Pt 2, pp. 86-93, 2018. View at: Publisher
Site
| PubMed
6. Florian E Buisman, Eric P van der
Stok, Boris Galjart, et al. “Histopathological growth patterns as biomarker for
adjuvant systemic chemotherapy in patients with resected colorectal liver
metastases.” Clin Exp Metastasis, vol. 37, no. 5, pp. 593-605, 2020.
View at: Publisher Site | PubMed
7. Boris Galjart, Pieter M H Nierop,
Eric P van der Stok, et al. “Angiogenic desmoplastic histopathological growth
pattern as a prognostic marker of good outcome in patients with colorectal
liver metastases.” Angiogenesis, vol. 22, no. 2, pp. 355-368, 2019. View
at: Publisher Site | PubMed
8. Wasswa William, Andrew Ware,
Annabella Habinka Basaza-Ejiri, et al. “A pap-smear analysis tool (PAT) for
detection of cervical cancer from pap-smear images.” Biomed Eng Online,
vol. 18, no. 1, pp. 16, 2019. View at: Publisher
Site
| PubMed
9. Ding-Qiao Wang, Long-Yu Feng,
Jin-Guo Ye, et al. “Accelerating the integration of ChatGPT and other
large-scale AI models into biomedical research and healthcare.” MedComm -
Future Medicine, vol. 2, no. 2, pp. e43, 2023. View at: Publisher Site
10. Dimitris Bertsimas, Georgios
Antonios Margonis, Suleeporn Sujichantararat, et al. “Using Artificial
Intelligence to Find the Optimal Margin Width in Hepatectomy for Colorectal
Cancer Liver Metastases.” JAMA Surg, vol. 157, no. 8, pp. e221819, 2022.
View at: Publisher Site | PubMed
11. R Ferrari, C Mancini-Terracciano, C
Voena, et al. “MR-based artificial intelligence model to assess response to
therapy in locally advanced rectal cancer.” Eur J Radiol, vol. 118, pp.
1-9, 2019. View at: Publisher Site | PubMed
12. Ming Y Lu, Bowen Chen, Drew F K
Williamson, et al. “A visual-language foundation model for computational
pathology.” Nat Med, vol. 30, no. 3, pp. 863-874, 2024. View at: Publisher
Site
| PubMed
13. Ginimol Mathew, Riaz Agha, Joerg
Albrecht, et al. “STROCSS 2021: Strengthening the Reporting of cohort,
cross-sectional and case-control studies in Surgery.” Int J Surg, vol.
96, pp. 106165, 2021. View at: Publisher
Site
| PubMed
14. Ming Y Lu, Drew F K Williamson,
Tiffany Y Chen, et al. “Data-efficient and weakly supervised computational
pathology on whole-slide images.” Nat Biomed Eng, vol. 5, no. 6, pp.
555-570, 2021. View at: Publisher
Site
| PubMed
15. Alexey Dosovitskiy, Lucas Beyer,
Alexander Kolesnikov, et al. “An image is worth 16x16 words: Transformers for
image recognition at scale.” Computer Vision and Pattern Recognition,
2020.
16. Julien Calderaro, Narmin Ghaffari
Laleh, Qinghe Zeng, et al. “Deep learning-based phenotyping reclassifies
combined hepatocellular-cholangiocarcinoma.” Nat Commun, vol. 14, no. 1,
pp. 8290, 2023. View at: Publisher
Site
| PubMed
17. Liu W, Lin Y, Liu Y, et al. “Vision
Transformer for Small-Size Datasets.” Neurocomputing, vol. 473, pp.
144-155, 2022.
18. Yunfang Yu, Wenhao Ouyang, Yunxi
Huang, et al. “AI-Based multimodal Multi-tasks analysis reveals tumor molecular
heterogeneity, predicts preoperative lymph node metastasis and prognosis in
papillary thyroid carcinoma: A retrospective study.” Int J Surg, 2024.
View at: Publisher Site | PubMed
19. Mathilde Caron, Hugo Touvron, Ishan
Misra, et al. “Emerging Properties in Self-Supervised Vision Transformers.” Proceedings
of the IEEE/CVF International Conference on Computer Vision (ICCV), pp.
9650-9660, 2021.
20. Zhuchen Shao, Hao Bian, Yang Chen,
et al. “TransMIL: Transformer based Correlated Multiple Instance
Learning for Whole Slide Image Classification.” 35th Conference on Neural
Information Processing Systems (NeurIPS 2021).
21. Yunyang Xiong, Zhanpeng Zeng,
Rudrasis Chakraborty, et al. “Nyströmformer: A nyström-based algorithm for
approximating self-attention.” Proc AAAI Conf Artif Intell, vol. 35, no.
16, pp. 14138-14148, 2021. View at: PubMed
22. Jie-Ying Liang, Shao-Yan Xi, Qiong
Shao, et al. “Histopathological growth patterns correlate with the immunoscore
in colorectal cancer liver metastasis patients after hepatectomy.” Cancer
Immunol Immunother, vol. 69, no. 12, pp. 2623-2634, 2020. View at: Publisher
Site
| PubMed
23. Diederik J Höppener, Pieter M H
Nierop, Joost Hof, et al. “Enrichment of the tumour immune microenvironment in
patients with desmoplastic colorectal liver metastasis.” Br J Cancer,
vol. 123, no.2, pp. 196-206, 2020. View at: Publisher
Site
| PubMed
24. Boris Galjart, Pieter M H Nierop,
Eric P van der Stok, et al. “Angiogenic desmoplastic histopathological growth
pattern as a prognostic marker of good outcome in patients with colorectal
liver metastases.” Angiogenesis, vol. 22, no. 2, pp. 355-368, 2019. View
at: Publisher Site | PubMed
25. Pulathis N Siriwardana, Tu Vinh
Luong, Jennifer Watkins, et al. “Biological and Prognostic Significance of the
Morphological Types and Vascular Patterns in Colorectal Liver Metastases
(CRLM): Looking Beyond the Tumor Margin.” Medicine (Baltimore), vol. 95,
no. 8, pp. e2924, 2016. View at: Publisher
Site
| PubMed
26. Kåre Nielsen, Hans C Rolff, Rikke L
Eefsen, et al. “The morphological growth patterns of colorectal liver
metastases are prognostic for overall survival.” Mod Pathol, vol. 27,
no. 12, pp. 1641-1648, 2014. View at: Publisher
Site
| PubMed
27. Vitoria Ramos Jayme, Gilton Marques
Fonseca, Isaac Massaud Amim Amaral, et al. “Infiltrative Tumor Borders in
Colorectal Liver Metastasis: Should We Enlarge Margin Size?” Ann Surg Oncol,
vol. 28, no. 12, pp. 7636-7646, 2021. View at: Publisher
Site
| PubMed
28. Pieter M H Nierop, Boris Galjart,
Diederik J Höppener, et al. “Salvage treatment for recurrences after first
resection of colorectal liver metastases: the impact of histopathological
growth patterns.” Clin Exp Metastasis, vol. 36, no. 2, pp. 109-118,
2019. View at: Publisher Site | PubMed
29. Hanna Nyström, Peter Naredi, Anette
Berglund, et al. “Liver-metastatic potential of colorectal cancer is related to
the stromal composition of the tumour.” Anticancer Res, vol. 32, no. 12,
pp. 5183-5191, 2012. View at: PubMed
30. Chiara Cremolini, Massimo Milione,
Federica Marmorino, et al. “Differential histopathologic parameters in
colorectal cancer liver metastases resected after triplets plus bevacizumab or
cetuximab: a pooled analysis of five prospective trials.” Br J Cancer,
vol. 118, no. 7, pp. 955-965, 2018. View at: Publisher
Site
| PubMed
31. Zhaoyang Xu, Carlos Fernádez Moro,
Danyil Kuznyecov, et al. “Tissue region growing for hispathology image
segmentation.” In: Proceedings of the 2018 3rd International Conference on
Biomedical Imaging, Signal Processing. Bari, Italy: Association for Computing
Machinery, 2018.
32. David Tellez, Diederik Höppener,
Cornelis Verhoef, et al. “Extending unsupervised neural image compression with
supervised multitask learning.” Proc Mach Learn Res, vol. 121, pp.
770-783, 2020.
33. David Tellez, Geert Litjens, Jeroen
van der Laak, et al. “Neural image compression for gigapixel histopathology
image analysis.” IEEE Trans Pattern Anal Mach Intell, vol. 43,no. 2, pp.
567-578, 2021. View at: Publisher
Site
| PubMed
34. Chiara Cremolini, Massimo Milione,
Federica Marmorino, et al. “Differential histopathologic parameters in
colorectal cancer liver metastases resected after triplets plus bevacizumab or
cetuximab: a pooled analysis of five prospective trials.” Br J Cancer,
vol. 118, no. 7, pp. 955-965, 2018. View at: Publisher
Site
| PubMed
35. Artem Shmatko, Narmin Ghaffari Laleh, Moritz Gerstung “Artificial intelligence in histopathology: enhancing cancer research and clinical oncology.” Nat Cancer, vol. 3, no. 9, pp. 1026-1038, 2022. View at: Publisher Site | PubMed